"I want to tell you that your fact sheet on the [Missile Technology Control Regime] is very well done and useful for me when I have to speak on MTCR issues."
Deterrence Under Uncertainty: Artificial Intelligence and Nuclear War
January/February 2025
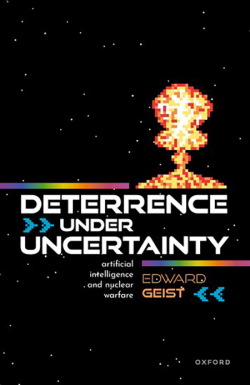
More Data Is Not the Answer
Deterrence Under Uncertainty: Artificial Intelligence and Nuclear War
By Edward Geist
Oxford University Press
2023
Reviewed by Herbert Lin
Some books are erudite and learned. Some books are entertaining and even funny. Some books offer a whole different perspective on a topic. Some books teach interesting things. Some books are well written. Deterrence Under Uncertainty by Edward Geist is exemplary in each of these categories. It is essential reading for a world awash with conversations about the revolutionary impact of artificial intelligence (AI) on armed conflict.
A bit of history is important to frame these conversations properly. In the 1970s, the Second Offset strategy was formulated as a way of using new technologies to counter the Soviet Union’s numerical superiority in conventional weapons.1 Investments initiated in this time frame continued through the 1980s, and the fruits of these investments were on display during the 1991 Persian Gulf War.
Responding to Iraq’s ground invasion of Kuwait in August 1990 and subsequent annexation of that Gulf state, the United States led an international coalition to eject Iraq from Kuwaiti territory and restore the latter’s sovereignty. Military operations against Iraqi forces in Kuwait began on January 17, 1991, with extensive aerial bombardments followed by a ground offensive on February 24.
Within 100 hours of the ground operation, a ceasefire was announced after coalition forces ejected Iraqi forces from Kuwait and then advanced into Iraqi territory. The use of precision-guided munitions; advanced command, control, computers, communications, intelligence, surveillance, and reconnaissance systems; and tactics enabled by these technologies resulted in remarkably low coalition casualties compared to previous conflicts. By contrast, Iraqi forces suffered significant losses, with tens of thousands killed or captured and vast amounts of military equipment destroyed. This swift victory underscored the effectiveness of the Second Offset strategy, which emphasized technological innovation to overcome numerical disadvantages on the battlefield.
In the wake of this war, many military decision-makers believed that a genuine revolution in battlefield awareness was closer than ever. For instance, Geist quotes U.S. Air Force Chief of Staff General Ronald Fogelman as saying in October 1996 that, “in the first quarter of the new century, it will become possible to find, fix or track, and target anything that moves on the surface of the earth.”2 Five years later, Vice Chairman of the Joint Chiefs of Staff Admiral William Owens suggested that the United States would “be able to see everything of military significance in the combat zone.”3
Lingering Ramifications
So it is today that the ramifications of the Second Offset are still felt as precision-guided munitions, delivered by stealth platforms and enhanced by global location awareness, continue to play a pivotal role in U.S. military strategy for engaging great powers in armed confrontation. These munitions, supported by advanced battlefield information systems communicating through U.S. Department of Defense networks, are intended to enable effective strikes against high-value targets even in heavily defended areas. Many of the platforms and munitions that would be used in such a conflict today are more advanced and sophisticated than those used in the Persian Gulf War, although many would be identical as well. Troops from that era would easily recognize the shape and character of many of today’s technology-enabled weapons if not their precise capabilities.
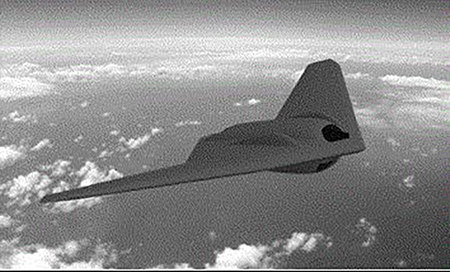
Military leaders and analysts are now pondering the significance of AI in this environment. In a key part of what reasonably could be called the Third Offset, that is, an AI-enabled Second Offset, the ability of AI to process vast amounts of battlefield data in real time is intended to provide commanders with an even more comprehensive situational awareness through the integration of diverse data sources, such as satellite and drone imagery, electronic warfare intercepts, and intelligence reports.
This information is combined with details about friendly forces, including where they are and what weapons they can bring to the fight, as well as environmental conditions, terrain, and the whereabouts of neutral actors or noncombatants. The resulting near-real-time situational awareness allows commanders to identify patterns and trends that might otherwise go unnoticed. This knowledge enables faster, more informed decision-making, which is essential in high-stakes environments where time is critical.
In this world, AI plays a critical role in a “God’s-eye view” of the battlefield, a concept rooted in the notion of “ground truth,” which denotes information gathered through direct observation and measurement. A God’s-eye view is utterly comprehensive, which means that it accounts for anything and everything that might be relevant to a commander’s decision-making. With a God’s-eye view, everything on the battlefield can be seen, what can be seen on the battlefield can be hit, and what can be hit can be killed. In this environment, victory soon follows.
Extending these capabilities for near-God-like battlefield awareness to the nuclear sphere does not take much imagination, given that senior military leaders have called for the seamless integration of nuclear and conventional command-and-control capabilities.4 Responding to such calls, analysts have raised concerns about the putative vulnerability of strategic nuclear systems.
Geist notes Rose Gottemoeller’s concerns that “[s]ecure retaliatory forces are becoming vulnerable…because ubiquitous sensing, paired with big data analysis [in context, an aspect of AI], makes it possible for adversaries to reliably detect those forces. Even moving targets, such as mobile missiles and submarines, may become vulnerable to detection and targeting.”5 He also notes Paul Bracken’s suggestion that “the long-prophesied epoch of splendid situational awareness is finally at hand because Al and deep learning will enable information fusion for data from many kinds of sensors, with a resulting ‘synergistic effect.’”6
Other analysts with similar concerns include James Johnson, who writes that “the integration of AI, machine learning, and big-data analytics can dramatically improve militaries’ ability to locate, track, target, and destroy a rival’s nuclear-deterrent forces—especially nuclear-armed submarines and mobile missile forces—and without the need to deploy nuclear weapons.”7 Johnson concludes that “the capabilities AI might enhance (cyber weapons, drones, precision-strike missiles, and hypersonic weapons), together with the ones it might enable (intelligence, surveillance, and reconnaissance, automatic target recognition, and autonomous sensor platforms), could make hunting for mobile nuclear arsenals faster, cheaper, and more effective than before.”
In a somewhat similar vein, other experts raise the possibility that AI may enable more effective targeting of mobile land-based systems,8 as the result of AI-enabled improvements in real-time surveillance coupled with a better understanding of the routines and doctrine that shape their dispersal patterns. Such improvements would take place through better processing of larger volumes of data from radar, satellite, and electronic sensors and the control of reconnaissance swarms of sensor platforms.
Geist’s Contribution
Into this conversation steps Geist. The heart of his contribution, a critically important one, is his insight that more data from sensors ultimately will not solve the problem, in this case, one of making now-survivable nuclear forces vulnerable to a splendid first strike. He notes that “[t]ime and again, when much-anticipated new computers and networks are placed into service, they fail to live up to expectations about their military effect.” Geist argues that “this pattern is not a coincidence, or the result of still-immature technology, but rather a straightforward implication of theoretical computer science” and that “‘lifting the fog of war’ is something that computers [no matter how powerful] cannot be counted upon to do.”
Why? The author offers two reasons. The first is relatively easy to understand: information that is received and integrated may be unreliable. As Geist points out,
“[I]ntelligence about troop movements might be ingenious disinformation planted by devious enemy agents; data about... missile tests might be distorted by faulty sensors; meteorologists might be drunk or simply incompetent.” Worst of all, people do not know what they do not know—the famous problem of “unknown unknowns.”
With such possibilities, it is not clear that more data will help. To make sense out of data of uncertain quality or reliability, one needs a working theory of which data to ignore or how much to downplay it. Any such theory is necessarily formulated outside the scope of the data available. If there were data available to help such formulation, that data itself would be of uncertain quality or reliability. Of course, if the theory of what data to ignore or downplay is wrong, the computation will be less reliable than it would be otherwise.
The second reason, which is independent of the first one, is rooted in a concept known as computational complexity. Sensors provide data from the battlefield, and from this data, AI is supposed to determine, which is to say compute, the specific reality that this data represents, yet there are many possible battlefield realities that would be consistent with the data in hand.
Many of these possible but incorrect realities can be eliminated if more data were available (e.g., if more sensors were available). In many cases, these possibilities would be eliminated because they would not be consistent with the enlarged set of data. As more data and constraints are added, however, the computational task of processing and analyzing this information also becomes more complex and demanding.
The complexity arises from the need to handle large volumes of data and the intricate task of synthesizing this information into a coherent, accurate picture of reality. Each new piece of data must be integrated and analyzed in conjunction with existing information, often requiring complex algorithms and models. This process can be computationally intensive because it involves balancing the volume of data with the capacity to process it effectively.
Ultimately, although more data can help improve understanding of the battlefield, it also increases computational demands. Advanced computational techniques and resources are required to manage and interpret large datasets accurately. Even worse, ingesting even small amounts of additional data increases the need for computing resources faster than these can be made available. Geist argues that having a God’s-eye level of battlefield awareness requires being able to compute a solution of very high complexity.
The most significant of the necessary computational resources is time. It is obvious that the planning needed to conduct a disarming strike against land-mobile missile launchers and ballistic missile submarines requires that the necessary computations be doable in a tactically relevant time. A computation that takes a billion years to complete is not useful, and the fact that such a computation is possible in principle is irrelevant for all practical purposes.
To translate the idea of computational complexity into lay terms, Geist provides a creative analog to navigating a bureaucracy with Kafka-esque rules for anyone who must and can sign off on various forms before some administrative approval for a particular action can be obtained. In this case, the problem to be solved is obtaining such approval, and one can see easily that, by adding more information requirements to the various forms that must be submitted, the longer it will take to review these forms and hence the longer it will take to solve the problem. In this bureaucracy, it sometimes happens that before an administrator can sign off on some form, another form needs to be created, asking the submitter for different information.
In some bureaucracies, this is a never-ending process and might result in the self-contradictory result that before the approval for a particular action can be obtained, the approval must already be in hand. Even if that is not the case, that in principle the approval for that action can be obtained without such a circular requirement and the clerks involved in the process work as fast as possible, skipping lunch and working overtime, it still may take centuries for the process to terminate.
Given such analysis, one might conclude that a computational approach to battlefield awareness is a hopeless task. Taking into account two factors, it may not be hopeless. First, the above result of computational intractability from complexity theory is true only for the “worst possible” battlefield scenario. There may well be many battlefield scenarios, what might be called average-case scenarios, where the performance of a system for battlefield awareness would be acceptable. The question then is whether the system designer can say safely that the on-the-scene commander will never face a worst-case battlefield scenario. Understanding the nature of this problem helps a great deal because domain-specific knowledge can be used to justify such a claim. Perhaps in practice, only average-case scenarios will arise, in which case the system providing battlefield awareness is a big win for the commander.
Second, although calculating optimal solutions may be computationally prohibitive, calculating approximate solutions, that is, solutions that are nearly optimal, often can require far fewer computational resources. “Nearly optimal” means that the solution calculated is guaranteed within a factor X of the optimal solution. X is known as the approximation ratio, and if X were known to be 2, the calculated “nearly optimal” solution would be within a factor of ½, or 2 of the unknown optimal solution. Tolerance for a less-than-optimal solution is what makes this approach acceptable.
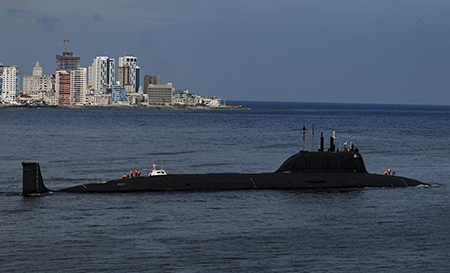
Unarguably, going down the path of approximate solutions entails some risk because it often involves making certain assumptions about the nature and structure of the problem. These assumptions are necessary to simplify the problem and enable the use of approximation algorithms that provide performance guarantees, such as specific approximation ratios. If those assumptions are wrong, the approximate solution calculated may be far from optimal, and the performance guarantee as described no longer will be valid. Yet, if system designers understand the problem well enough to make good simplifying assumptions, the system for battlefield awareness is again a win for the commander.
AI and Strategic Stability
Finally, Geist addresses a vitally important point omitted in most analyses of how AI might affect strategic stability. It is true that if states with nuclear weapons continue to design and operate their nuclear strategic forces the way they do today while technology continues to advance, they could start becoming vulnerable, but it is absurd to believe that these states will not do everything they can to reduce such vulnerabilities. Even if Nation A deployed a plethora of surveillance and reconnaissance systems to find the land-mobile missile launchers or the ballistic missile submarines of Nation B and even if A had the computational capabilities and resources to integrate volumes of data that are orders of magnitude larger than those that are flowing today, it is not credible that B would act passively and allow its second-strike systems to lose their ability to evade attack.
Instead, it would make much more sense for Nation B to deploy active and passive countermeasures to frustrate Nation A’s attempts to compromise its second-strike forces. B has the advantage over A in that B generally knows the capabilities and vulnerabilities of its own systems better than A and thus, with its own attack simulators, can test the effectiveness of a variety of countermeasures it could take against A’s threatened attack. A, not knowing B’s systems or countermeasures nearly as well as B, would be planning an attack without the benefit of knowing B’s responses. Indeed, Geist argues that AI would be most useful for B in developing and deploying its countermeasures.
This experience has been repeated again and again. When ballistic missiles became vulnerable to anti-ballistic missile systems, the operators of ballistic missiles developed multiple independently targetable reentry vehicles and penetration aids. When radar stripped away the cloak of invisibility for bombers and fighter planes, the operators of bombers and fighter planes deployed chaff and jamming technologies and flew low rather than high. In the future, as land-mobile intercontinental ballistic missile launchers or ballistic missile submarines become more detectable when operationally deployed, decoys, active sensor countermeasures, and other responses are sure to follow.
This book should be required reading for every strategic analyst. It appears to be the only published work outside the domain of the technical scientist or engineer that links the implications of new information processing technologies for battlefield awareness to the deep insights available through theoretical computer science.
It is tempting to assume that continuing exponential growth (the often-invoked Moore’s law9) in the capabilities of that technology will cover all computational contingencies. Yet, theoretical computer science, by comparison quite unfamiliar to most strategic analysts, clearly demonstrates the relationship between integrating more data inputs and increasing computational requirements. Geist’s short if densely argued treatise is a basic introduction to some themes in theoretical computer science that are as important to today’s strategic analyst as a familiarity with Moore’s law.
ENDNOTES
1. Rebecca Grant, “The Second Offset,” Air Force Magazine, July 2016.
2. See John A. Tirpak, “Find, Fix, Track, Target, Engage, Assess,” Air Force Magazine, July 2000.
3. See William Owens and Ed Offley, Lifting the Fog of War (Baltimore: Johns Hopkins University Press, 2001), p. 96.
4. For example, Air Force Lieutenant General James Dawkins, deputy chief of staff for strategic deterrence and nuclear integration, has said that “[w]e’ve got to have [a nuclear command, control and communications system] Enterprise Architecture that provides [nuclear command and control] over assured comms for seamless integration of conventional and nuclear forces.” Theresa Hitchens, “Congress Fears DoD Not Prepared for NC3 Cyber Attacks,” Breaking Defense, December 11, 2020.
5. See Rose Gottemoeller, “The Standstill Conundrum: The Advent of Second-Strike Vulnerability and Options to Address It,” Texas National Security Review, Vol. 4, No. 4 (2021): 115-124.
6. Paul Bracken, “The Hunt for Mobile Missiles: Nuclear Weapons, Al, and the New Arms Race,” Foreign Policy Research Institute, September 2020, p. 98.
7. James Johnson, “Rethinking Nuclear Deterrence in the Age of Artificial Intelligence,” Modern War Institute, January 28, 2021(emphasis in original).
8. Michael Klare and Xiaodon Liang, “Beyond a Human ‘in the Loop’: Strategic Stability and Artificial Intelligence,” Arms Control Association Issue Brief, November 12, 2024.
9. Data on semiconductor manufacturing indicate that Moore’s law, when framed in terms of an exponentially declining cost per transistor on a chip, is reaching the end of its useful lifetime. The actual cost per transistor began to level off around 2012, and it has followed Moore’s law predictions since then. See “Semiconductors,” in The Stanford Emerging Technology Review 2023: A Report on Ten Key Technologies and Their Policy Implications, ed. Herbert S. Lin, 2023.
Herbert Lin is a senior research scholar and the Hank Holland Fellow at Stanford University.